Strategic Allocation
Is there a best way to select and weight asset classes for long-term diversification benefits? These blog entries address this strategic allocation question.
May 11, 2023 - Size Effect, Strategic Allocation, Value Premium
Can investors improve long-term retirement portfolio outcomes by targeting equity factor premiums in their stock allocations? In his April 2023 paper entitled “How Targeting the Size, Value, and Profitability Premiums Can Improve Retirement Outcomes”, Mathieu Pellerin investigates whether stock portfolios that target size, value and profitability factor premiums better sustain retirement spending and generate larger bequests than those holding the broad stock market. His hypothetical investor:
- Starts saving at 25, retires at 65 and dies at 95.
- Initially allocates 100% to stocks, at age 45 reduces this allocation linearly to 50% at age 65 by shifting to bonds, and thereafter maintains 50%/50% stocks/bonds.
- Makes $1,042 monthly contributions ($12,500 per year, or $500,000 from age 25 to 65).
- After retirement, withdraws (consumes) a constant annual 4% in real terms of the balance at retirement.
- For the stock allocation, chooses either a broad value-weighted market index (CRSP 1-10) or the Dimensional US Adjusted Market 1 index that emphasizes size, value and profitability factors with low turnover.
- Earns real annual broad stock market returns of either 8.1% (actual historical average) or 5.0% (a conservative 5th percentile of historical return distribution).
- For the bond allocation, holds 5-year U.S. Treasury notes.
He simulates 100,000 lifecycles by, for each lifecycle: (1) extracting 70-year (840-month) real asset class return subsamples from the full histories; and, (2) applying block bootstrapping with 10-year mean block size to generate lifecycle portfolio returns. Using monthly historical returns for the specified stock/bond proxies and monthly U.S. inflation data during June 1927 through December 2022, he finds that:
Keep Reading
April 28, 2023 - Momentum Investing, Strategic Allocation, Technical Trading
A subscriber asked about the performance of a variation of the monthly reformed 50-50 Simple Asset Class ETF Value Strategy (SACEVS) Best Value-Simple Asset Class ETF Momentum Strategy (SACEMS) Equal-Weighted (EW) Top 2 combination that substitutes 100% SACEMS EW Top 2 whenever both:
- SPDR S&P 500 ETF Trust (SPY) is the selection for SACEVS Best Value at the end of the prior month.
- SPY is below its 10-month simple moving average at the end of the prior month.
The objective of the variation is to suppress SACEVS Best Value drawdowns. To investigate, we compare performance results for this variation (“Filtered”) with those for baseline 50-50 SACEVS Best Value-SACEMS EW Top 2. Using monthly returns for SACEVS Best Value and SACEMS EW Top 2 since July 2006 (limited by SACEMS) and monthly dividend-adjusted prices for SPY since September 2005, all through March 2023, we find that: Keep Reading
April 24, 2023 - Calendar Effects, Strategic Allocation
A subscriber asked whether the Simple Asset Class ETF Momentum Strategy (SACEMS) exhibits monthly calendar effects. In investigating, we also look at the Simple Asset Class ETF Value Strategy (SACEVS)? We consider the Best Value (most undervalued asset) and Weighted (assets weighted by degree of undervaluation) versions of SACEVS. We consider the Top 1, equal-weighted (EW) Top 2 and EW Top 3 versions of SACEMS, which each month holds the top one, two or three of nine ETFs/cash with the highest total returns over a specified lookback interval. We further compare seasonalities of these strategies to those of their benchmarks: for SACEVS, a monthly rebalanced 60% stocks-40% bonds portfolio (60-40); and, for SACEMS an equal-weighted and monthly rebalanced portfolio of the SACEMS universe (EW All). Using monthly gross total returns for SACEVS since August 2002 and for SACEMS since July 2006, both through March 2023, we find that:
Keep Reading
April 20, 2023 - Gold, Strategic Allocation, Volatility Effects
Does an allocation to gold truly protect a portfolio from downside risk? In their April 2023 paper entitled “The Golden Rule of Investing”, Pim van Vliet and Harald Lohre examine downside risks for portfolios of stocks (value-weighted U.S. stock market) and bonds (10-year U.S. Treasury notes) with and without gold (bullion) based on real returns and a 1-year investment horizon. They also investigate substitution of low-volatility stocks for the broad stock market in search of further downside risk protection. Using monthly returns for the specified assets and U.S. inflation data during 1975 (when gold becomes truly tradable) through 2022, they find that:
Keep Reading
March 31, 2023 - Strategic Allocation
A subscriber asked about backtesting two alternatives to equal weight (EW) for the Simple Asset Class ETF Momentum Strategy (SACEMS) Top 2 and Top 3 portfolios, as follows:
- Fixed Weighted:
- For Top 2, 66% allocation to the first-place exchange-traded fund (ETF) and 34% to the second-place ETF.
- For Top 3, 50% allocation to the first-place ETF, 30% to the second-place ETF and 20% to the third-place ETF.
- Variable Weighted: compute allocations based on ratios of momentum ranking interval returns.
To investigate, we compare SACEMS gross performance statistics of the alternative weighting schemes with those of the baseline EW scheme. For variable weights, if the past return of the third-place ETF is negative, we ignore it in Top 3 calculations. If the past return of the second-place ETF is negative, we ignore it in Top 2 calculations (allocating 100% to the first-place ETF, which never has negative past return). Using SACEMS input data through February 2023, we find that: Keep Reading
March 24, 2023 - Equity Options, Strategic Allocation
Does the difference in individual stock/market return relationships between good times (relatively low correlations) and bad times (relatively high correlations) present an easy and efficient way to hedge against stock market crashes (tail risk)? In their March 2023 paper entitled “Tail Risk Hedging: The Search for Cheap Options”, Poh Ling Neo and Chyng Wen Tee test the ability of a portfolio of liquid but cheap put options on individual stocks to protect against equity market crashes. They reason that:
- These options are inexpensive compared to equity index put options.
- During good times, the relatively low return correlations across stocks limit option portfolio drag.
- During market crashes, the spike in these correlations confers on the option portfolio tail risk protection comparable to that of equity index put options.
Their tests encompass three stock market regimes: (1) up months have positive monthly returns and no daily return less than -5%; (2) down months have negative monthly returns but no daily return less than -5%; and, (3) tail risk months have at least daily return less than -5%. At the end of each month, they construct a crash protection put option portfolio as follows:
- Select an out-of-the-money put option for each optionable stock with delta closest to -10% and six months to a year until expiration.
- Exclude those with ex-dividend dates prior to expiration.
- Exclude those with bid-ask spreads over 50% of the bid-ask midpoint.
- Allocate 2% of the value of the S&P 500 Index position equally to each of the cheapest 20% of remaining put options.
Most analyses assume option buys and sells occur at bid-ask midpoints (no frictions), but they do look at impacts of effective bid-ask spreads up to 50% of the quoted spread. Using daily returns for the S&P 500 Index, S&P 500 Index put options and individual U.S. stock put options during January 1996 through December 2020, they find that: Keep Reading
March 23, 2023 - Fundamental Valuation, Momentum Investing, Strategic Allocation, Technical Trading, Volatility Effects
A subscriber asked about boosting the performance of the Simple Asset Class ETF Value Strategy (SACEVS) and the Simple Asset Class ETF Momentum Strategy (SACEMS), and thereby the Combined Value-Momentum Strategy (SACEVS-SACEMS), by substituting ProShares Ultra S&P500 (SSO) for SPDR S&P 500 ETF Trust (SPY) in these strategies whenever:
- SPY is above its 200-day simple moving average (SMA200); and,
- The CBOE Volatility Index (VIX) SMA200 is below 18.
Substitution of SSO for SPY applies to portfolio holdings, but not SACEMS asset ranking calculations. To investigate, we test all versions of SACEVS, SACEMS and monthly rebalanced 50% SACEVS-50% SACEMS (50-50) combinations. We limit SPY SMA200 and VIX SMA200 conditions to month ends as signals for next-month actions (no intra-month changes). We consider baseline SACEVS and SACEMS (holding SPY as indicated) and versions of SACEVS and SACEMS that always hold SSO instead of SPY as benchmarks. We look at average gross monthly return, standard deviation of monthly returns, monthly gross reward/risk (average monthly return divided by standard deviation), gross compound annual growth rate (CAGR), maximum drawdown (MaxDD) and gross annual Sharpe ratio as key performance metrics. In Sharpe ratio calculations, we employ the average monthly yield on 3-month U.S. Treasury bills during a year as the risk-free rate for that year. Using daily unadjusted SPY and VIX values for SMA200 calculations since early September 2005 and monthly total returns for SSO since inception in June 2006 to modify SACEVS and SACEMS inputs, all through February 2023, we find that: Keep Reading
March 16, 2023 - Equity Premium, Investing Expertise, Strategic Allocation
Can machine learning perfect equity sector rotation? In the January 2023 version of their paper entitled “Deep Sector Rotation Swing Trading”, flagged by a subscriber, Joel Bock and Akhilesh Maewal present a sector rotation strategy guided by multiple-input, multiple output deep learning model. The strategy chooses weekly from among 11 U.S. sectors using exchange-traded fund (ETF) proxies. Specifically, each week during each year, they:
- Train the machine learning model on the last two years of weekly (Friday close) historical sector ETF prices and volumes and sometimes auxiliary economic data (10-year U.S. Treasury yield, USD currency index, crude oil proxy and stock market volatility) to predict next-week opening and closing prices for each ETF.
- Compare the predicted return estimate for each ETF to a dynamically updated threshold return to screen for potential buys.
- Apply additional filters to screen out potential buys with unusual past losses to accommodate investor loss aversion.
- At the next-week open, allocate available capital to surviving sector ETFs based on respective past win rate (profitable trade) and respective past sector trade momentum.
- Liquidate all positions just prior to the next-week close.
Their benchmark is buying and holding the S&P 500 Index with reinvested dividends. Using weekly inputs as described during January 2012 through December 2022, they find that:
Keep Reading
March 13, 2023 - Bonds, Strategic Allocation
“Simplified Offensive, Defensive and Risk Mode Identification Momentum Strategy” describes a strategy that each month holds offensive (defensive) assets when average return on iShares TIPS Bond ETF (TIP) over the past 1, 3, 6 and 12 months is positive (negative). Is past return of TIP, which purely impounds investor expectations for U.S. inflation, a reliable indicator of future asset class returns? To investigate, we relate TIP returns to future returns for each of the following exchange-traded fund (ETF) asset class proxies:
- Equities:
- SPDR S&P 500 (SPY)
- iShares Russell 2000 Index (IWM)
- iShares MSCI EAFE Index (EFA)
- iShares MSCI Emerging Markets Index (EEM)
- Bonds:
- iShares Barclays 20+ Year Treasury Bond (TLT)
- iShares iBoxx $ Investment Grade Corporate Bond (LQD)
- iShares JPMorgan Emerging Markets Bond Fund (EMB)
- Real assets:
- Vanguard REIT ETF (VNQ)
- SPDR Gold Shares (GLD)
- Invesco DB Commodity Index Tracking (DBC)
We consider both linear correlation and non-linear ranking tests. We look at TIP returns over the past 1, 3, 6 and 12 months separately, and as an average of these past returns (average past TIP return). Using monthly dividend-adjusted returns for TIP and the above asset class proxies as available during December 2003 (limited by TIP) through February 2023, we find that: Keep Reading
February 23, 2023 - Strategic Allocation, Volatility Effects
Is portfolio downside risk better manageable by combining drawdown and recovery into a single “submergence” metric? In their February 2023 paper entitled “Submergence = Drawdown Plus Recovery”, Dane Rook, Dan Golosovker and Ashby Monk present submergence density as a new risk metric to help investors analyze asset/portfolio drawdown and recovery jointly. They define submergence (s) of an asset/portfolio as percentage of current value below its past highwater mark. They define submergence density (d) during a given sample period as the κ-root of the sum of measurement interval submergences each raised to the κ-power during the sample period, as follows:
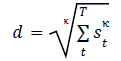
When κ=1, submergence density is arithmetic average drawdown. When κ=∞ (or a very high number), submergence density is maximum drawdown. They use κ = 5 in examples to represent typical investor drawdown sensitivity. They define submergence risk-adjusted return as excess return (relative to the risk-free rate) minus a fraction (Θ) of submergence density, using the range 0.2 to 0.5 as reasonable for Θ. They apply submergence to several market indexes and discuss portfolio diversification and rebalancing in the context of reducing submergence overlaps. Using monthly excess returns for U.S. stocks, corporate bonds and Treasuries (S&P 500 Index, ICE BoA Corporate Bond Index and Bloomberg Treasuries Index, respectively) during 1979 through 2022, they find that:
Keep Reading